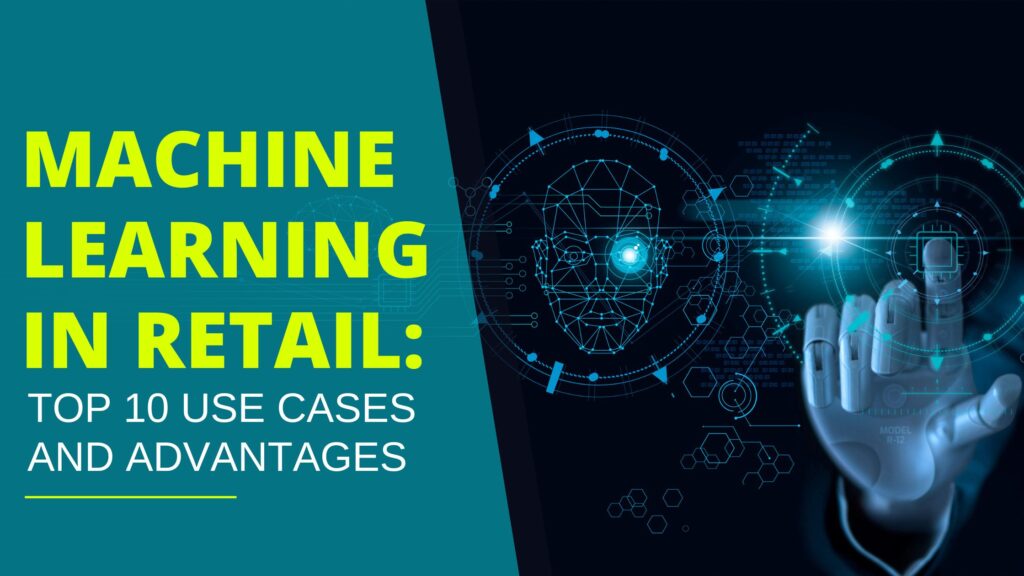
Artificial intelligence is gaining a high level of urgency for organizations willing to remain relevant in the current dynamic retail market. When current day retailers are adapting to this digital change, they are employing these sophisticated technologies aided by machine learning consultants. It helps enhance customer experiences, optimize operations and boost profitability. This blog post explores the top 10 use cases of machine learning in retail and highlights the key advantages of integrating ML into real strategies. Come, let us have a look.
Applications of Machine Learning in Retail
Machine learning can be of great use to retail in many different ways. Those are revolutionizing the industry by boosting the decision-making processes and enriching the customer experiences. ML makes the retailers capable of predicting the trend and make other operations that even cut down the cost to a greater extent. Through these developments, the retailers remain relevant and reduce their inability to meet the needs of the consumers in the current society.
Sales Forecasting Using Predictive Analytics
An effective way of decision making, predictive analytics, relies on statistical models. These employ machine learning techniques to analyze historical data to predict future events. Because trends in the data on previous sales are clearly seen, retailers are better at predicting demand. It also avoids problems like lack of stock or overstocking which causes a lot of trouble for a business. Overall, it enhances the companies’ sales and results in cutting down its expenses. As per the findings of the recent market survey, it has been revealed that retail organizations can expect good returns of up to 10-15% when predictive analytics are being used.
Personalized Marketing and Recommendations
There are several ways that machine learning efforts are being used in retail, with one of the most prevalent being the ability to deliver personalized marketing. A company uses the information that the customer had been using while making the purchase, the customer’s browsing history, and their demographic information to recommend products to them. It also increases conversion by up to 20% since customers respond better to promotions targeted at them.
Optimization of Inventory
It is very important to ensure that there is an appropriate stock of products to avoid either having excess or none at all. Information from sales records, the time when certain products are most popular, and customer purchasing behavior is used by machine learning algorithms to set the most appropriate stock volume. This helps in the management of warehousing space, and also guarantees continual stock for some of the market demands. Companies that use ML in the management of inventory have reported a 30% cut on the number of stockouts.
Price Optimization
Artificial intelligence tools assist retailers in determining the prices that fluctuate based on the competitors’ prices, demand, and customer’s price sensitivity. This in a way allows retailers to get the best return on their investment while at the same time ensuring they continue to meet or exceed the expectations of their clientele. Indeed, the companies that have adopted pricing models based on ML have reported better profit margins that have increased dynamically.
Efficiency Improvement of Fraud Detection and Prevention
Because of the constantly progressing online economy, fraud prevention is a major concern in the retail industry. One more checkbox that is checked by machine learning algorithms is the identification of such unusual activities as dramatic increase of purchase frequency or frequent credit card usage. A number of reliable sources have noted that using deep machine learning for fraud detection decreases the incidence of fraudulent transactions for retailers by up to 50% saved by retailers.
Customer Sentiment Analysis
Machine learning in retail allows firms to analyze customer reviews, comments at social media, and feedback. By using positive, negative and neutral feelings, the level of satisfaction of the customers can be measured, and retailers can adapt accordingly. For instance, if the algorithm recognizes that there are growing negative feelings towards a particular product, then a brand is swift to tackle the problems that were raised.
Virtual Shopping Assistants
Virtual shopping powered by machine learning helps customers through the provision of real time help from virtual shopping assistants. Chatbots and voice assistants respond to queries, provide users with navigation and direction within products, and can assist with a purchase. More and more customers prefer self-service tools which reveal that virtual assistants really help in improving efficiency of online support services.
Shelf Management with Computer Vision
Computer vision, a branch of AI, helps retailers maintain an organized and well-stocked shelf display. Cameras and image recognition algorithms identify low stock levels or misplaced items. It ensures that shelves are always in perfect order. This real-time visibility allows employees to quickly restock, improving both efficiency and customer experience.
Demand Forecasting for Seasonal Trends
In retailing, there is normally a shift of aid at a certain point in the year and it is important to be able to forecast these changes. Mathematical models can be applied to historical sales records and external conditions including the weather, the economic climate, and social culture. Through planning demand during the holiday seasons or the peak seasons, the retailers will likely be in a position to respond to changes so as to maximize the sales and minimize on stock.
Return Management and Customer Service Improvement
Managing returns is a challenge for most retailers, especially with online shopping. ML analyzes patterns in return behavior and identifies potential causes, such as product quality or inaccurate sizing. Retailers then address these issues to reduce returns and enhance customer satisfaction. Additionally, machine learning-powered customer service systems enable faster response times and personalized support.
Advantages of Implementing Machine Learning in Retail
The benefits of machine learning in retail extend beyond just operational efficiency. Here are some key advantages:
Cost Savings
Machine learning for inventory control and driving prices for maintaining optimum stock and avoiding too much waste decrease the costs that result from the accumulation of surplus stock or input pricing below cost.
Enhanced Customer Satisfaction
Customized suggestions and enhanced customer services would satisfy consumers and hence enhance the shopping experience.
Reduced Fraud and Risks
Reduction of fraudulent losses, that is, maximum fraud prevention leads to high customers’ confidence.
Improved Decision-Making
Through valuable insights, customers were able to make the right decision that was in line with their needs, as assisted by machine learning.
Conclusion
Machine learning is reshaping the retail industry and offers numerous benefits from personalized customer experiences to enhance security and operational efficiency. As technology continues to evolve, the potential applications and advantages of machine learning in retail will only expand. Retailers that embrace these innovations will be well positioned to grow in an increasingly competitive market. Machine learning consultants play a crucial role in helping retailers adopt these advanced technologies. Consultants assist in selecting the right algorithms, setting up data infrastructure, and ensuring the successful implementation of machine learning models.