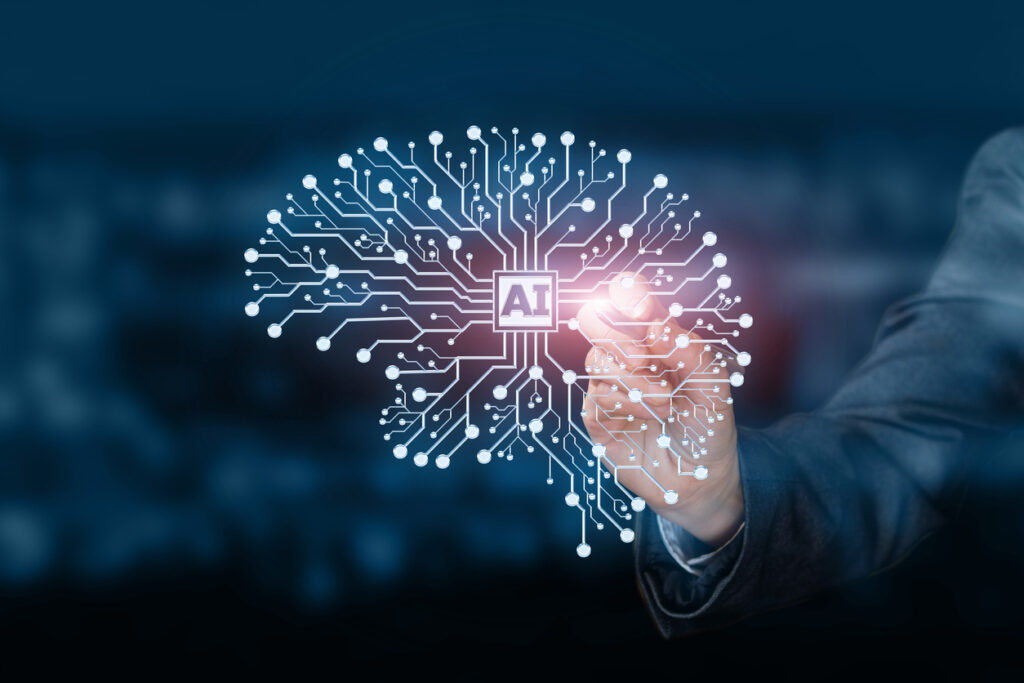
These days, AI-based predictive analysis isn’t just a new technology or yet another buzzword thrown around in business digital transformation services. Predictive analytics has come of age; it is a useful tool for any progressive organization not just to survive but thrive in today’s turbulent and rapid-paced, genuinely competitive business environment. The ability to drive data-informed decisions that forecast next events, trends, or customer behaviors creates whole industries, optimizes business processes, and produces better results.
Businesses relying on conventional, reactive strategies can thus only play catch-up in such dynamic markets, where every new development outside the walls of their company seems to call for a response rather than an ability to sail their ship in a predetermined direction. That is, however, precisely where predictive analytics comes in for critical advantage, especially with AI at the tiller. Transforming raw data into strategic insights means that businesses can create anticipation about and adapt to trends well before these show into the competitive view. Besides affording organizations an edge, the same empowers them to make decisions that are smarter, quicker, and faster.
Anticipating Trends With AI-Powered Predictive Analytics
In any industry, being able to anticipate future trends is paramount. By monitoring consumer demand patterns, shifts in the market, and minor/major operational disruptors, being able to see around the corner and respond in proactive terms empowers businesses to operate more effectively. Artificially intelligent predictive analytics enables businesses to tap into large amounts of historical and real-time data, thus predicting the occurrence of an event in the future with considerable accuracy.
1. Market Trends and Demand Forecasting
AI-powered predictive analytics changes the ball game in demand forecasting, especially for consumer-driven industries such as retail. Conventionally, demand forecasts have been cumbersome and usually full of errors based on historical sales data and simple extrapolations of trends. However, using business analytics, one can process complex sets of data like social media mentions, customer sentiment analysis, competitive pricing, and weather patterns coupled with macroeconomic data to make far more precise predictions.
For instance, within the fashion industry, AI can go through analysis on social media trends, influencer activity, search behavior, and even images shared online to predict which styles and colors are likely to dominate the next season. AI-powered tools like IBM’s Watson analyze all these external factors and cross-refer them with historical sales data to forecast demand for particular clothing items. This will enable them to optimize inventory and avoid costly overproduction or stockouts.
2. Prediction of Consumer Behaviour and Preference
AI is heavily used in capturing consumer behavior and preference. With the power of ML, NLP, and reinforcement learning, businesses can predict every behavior of their customers with ease. The prediction is also much more precise compared to traditional techniques of segmentation, as these depend on incomparably richer datasets that include online interactions, past purchases, browsing habits, and even psychological and demographic profiles.
For example, Netflix uses AI to predict what content would interest each of its users individually, making the Netflix viewing experience highly personalized. For instance, analyzing past user behavior and interaction-even down to genre, or time of day at which that content was consumed-Netflix issues real-time recommendations with the intent of retaining user engagement and reducing churn.
Automakers are also using AI to try and predict how consumer-buying behavior is increasingly changing with interest in electric vehicles. Through analyzing the trend of sales, changes in regulation, and environmental concerns, they know when a demand for EVs can be expected and will make necessary adjustments in production, supply chains, and marketing strategies accordingly.
Optimizing Resources With AI-Powered Predictive Analytics
The modern resource-constrained scenario urges resource optimization to maximum efficiency, elimination of waste, and competitive advantage. AI-driven predictive analytics also leverages resource optimization through better demand forecasting, inventory management, and predictive maintenance.
1. Inventory Management Optimization
AI-powered predictive analytics is revolutionizing the way retail and manufacturing companies manage their product levels. For instance, companies will be in a position to estimate fluctuations in demand, optimize stock level, and minimize the costly problem of overstocking or understocking.
For example, Amazon reinforces AI-powered predictive analytics to forecast the demand for products at a highly granular level to optimally use their wide network of fulfillment centers. By using predictive models processing data on sales, supply chain logistics, and even weather patterns, Amazon ensures that the hyper chosen products are in the right place at the right time, thereby minimizing storage costs and increasing customer experiences.
2. Predictive Maintenance in Manufacturing
AI-powered predictive maintenance systems further streamline their processes in terms of manufacturers dealing with equipment. Therefore, it does not depend on scheduled equipment maintenance, which often results in either excessive or too little downtime, but rather is predicted ahead of time by the analysis of an AI system when it is fed data coming in real-time from sensors.
Take the case of General Electric (GE), applying AI to predict part failure within its industrial equipment. By embedding sensors in machines, which continuously monitor certain variables such as temperature, vibration, and pressure, for example, AI systems from GE predict where a component is likely to fail. This enables them to carry out just-in-time maintenance, reducing unexpected downtime and prolonging equipment life to save on enormous costs associated with reactive repairs.
3. Optimization of Workforce Management
AI-powered predictive analytics is also evolving how businesses optimize labor resources. For example, airlines use predictive analytics to optimize flight crew scheduling by factoring in variables such as weather disruptions, flight delays, and consumer demand for passenger airliners. With this kind of prediction of staffing needs, airlines reduce operational costs while ensuring conformity to regulations.
Accordingly, AI models in a field such as healthcare enable hospitals to forecast the volumes of persons in need on treatment grounds, thereby informing human resource management planning for predictable demand in staffing. In that case, predictive analytics can anticipate times when EDs are most peak, thus ensuring appropriate staffing numbers that would handle surges in patients without unnecessary overtime hours in less busy times associated with higher staffing.
Proactive Decide-Making Using AI-powered Predictive Analytics
The most valuable output of AI-driven predictive analytics, perhaps, is the ability to act proactively without merely responding to events as they unfold. In other words, predictive models afford organizations the opportunity to take proactive measures with a view to minimizing risks, capitalizing on opportunities, and leading their strategy in alignment with future market conditions.
1. Reducing Financial Risk
Predictive analytics finds an increased application in the financial services industry for the evaluation of risks and opportunities. AI-driven predictive models are employed in financial institutions for the analysis of credit risks, prediction of loan defaults, and other hints of financial fraud.
For example, it could be the use of AI at LendingClub and in other FinTech companies to assess the creditworthiness of a borrower by taking into consideration data on this person’s credit score, transactions, and even social behavior. Therefore, with predictability provided by machine learning, such models can approximate the likelihood of a borrower’s state of default and, correspondingly, make it possible for lenders to hedge against this result using increased interest rates or by totally refusing to give loans to high-risk borrowers.
In the same manner, investment companies make use of artificial intelligence models when analyzing a broad base of real-time market data in making predictions, either on the fluctuation of stock prices or on economic condition changes. AI models are able to remove noise from market sentimental analysis, macroeconomic variables, geopolitical events, and many other factors to help an investor make a proactive investment decision to mitigate risk.
2. Healthcare Proactive Care
Predictive analytics is letting the healthcare organization move from reactive to proactive care delivery. AI models identify probable outcomes of the patient, early warning signals for disease progression, and suggest preventive measures in advance.
For example, AI-powered tools-such as DeepMind’s predictive models-are already applied by doctors to predict serious conditions, including AKI, allowing medical professionals to intervene early with hopes of saving lives and reducing healthcare costs.
The other side of the coin is predictive analytics for patient demand and resource allocation by hospitals and healthcare providers. Predictive modeling of patient volume, whether in the emergency department or at inpatient units, can allow hospitals to enhance resource utilization with regard to staffing quite quickly and render timely care, thus improving efficiency in patient outcomes and operational processes.
Competitive Advantage Through AI-Driven Predictive Analytics
The power of AI-driven predictive analytics can help organizations sustain a compelling competitive advantage in dynamic markets. Some ways AI does this are as follows:
- Faster Decision-Making: AI analytics enables the company to make its decisions faster and more ‘on target’, faster, and with near-instant insights. Thanks to its capability in voluminous real-time data processing, such organizations can become much quicker in reacting to changes hitting the market and immediately adjust their strategies before any other competitor even knows what is going on.
- Improved Customer Experience: It grants companies huge insight into customer preferences and behaviors to enable personalization at scale, thus delivering offerings that are more tailored, improving customer loyalty. By being able to anticipate what their customers will need, they can optimize their offerings for improved retention and value.
- Proactive Resource Management: AI helps businesses predict the need for resources reputedly and proactively allocates them. That would mean operations are always optimized, inefficiencies reduced, and costs cut towards more profitability.
- Minimized Risks and Costs: Predictive models reduce risks and costs by helping to identify potential risks before they become actual. This will give the businesses the opportunity for early action, thus reducing or avoiding entirely any chances of unexpected augmentation of costs due to things like equipment failure or market downturns.
Conclusion
AI-driven predictive analytics is no doubt a transformational technology in the digital era. This technology helps business enterprises to look ahead, optimize the available resources, and take decisions well in advance, thus enhancing operational efficiency and enhancing the key competitive advantage. With increased AI adoption in an organization’s strategic decision-making process, the insights that predictive analytics provide will become a key determinant for innovation, growth, and leadership of the organizations in their relevant markets.
FAQs
1. How Does AI-powered Predictive Analytics Work?
It uses machine learning and statistical models in concert with historical data to predict future events or behavior. Predictive analytics driven by AI scans the pattern in data and then makes suggestions, improving over time, always learning from new inputs.
2. Within which sectors of industries does AI-based Predictive Analytics find most applications?
The different industries that equally use predictive analytics with all its powerful features include healthcare, finance, retail, manufacturing, and transportation-all those bases that have much to do with complex data and fast, accurate decisions.
3. Will predictive analytics powered by AI replace human decision-making?
It can support and augment human decision-making, never fully replacing it. While the AI provides predictive insights, human expertise is required for interpreting results and making nuanced decisions.
4. How correct are the predictions of AI?
The quality and quantity of the data, added to the complexity of the predictive model, express the accuracy of the predictions made by AI. If this data is clean and comprehensive, the AI models can then make very accurate forecasts that sometimes even surpass those from traditional methods.
5. In what ways can predictive analytics enhance customer experience?
It enables one to do predictive analytics, where a company can predict customers’ needs and personalize interactions; thereafter, it can make targeted offers or render services to improve customer satisfaction and retention.
6. What does Machine Learning have to do with Predictive Analytics?
With machine learning, the predictive analytics models become capable of automatic improvement with time, as they learn from incoming data without explicit programming. It makes the predictions more accurate and adaptive.