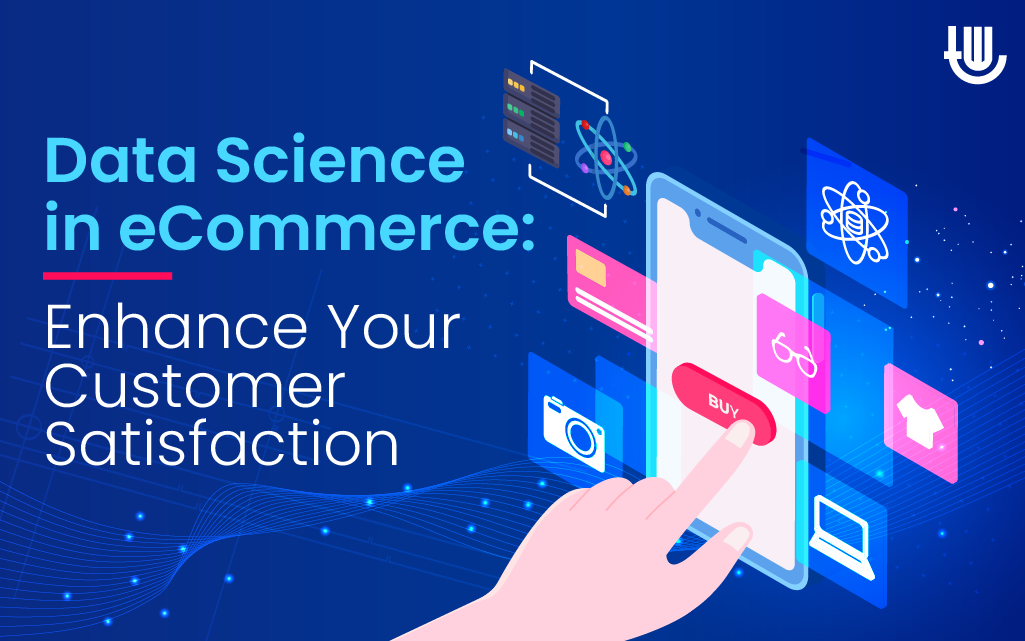
Data science in eCommerce plays a crucial role in improving customer satisfaction. As the number of online store users grows, businesses must deal with an overwhelming volume of data. Thus, extracting meaningful insights has become challenging. As a result, organizations might struggle with adapting to the ever-changing market environment.
Companies can better understand their clients’ needs and preferences using data science. They can simplify the process of identifying demand changes and forecasting sales. Also, they can provide users with a personalized approach based on the generated data.
In this article, we will highlight the influence of data science on eCommerce. Be ready to explore the use cases that your business can adopt today.
Impact of Data Science on Customer Service
Data science in eCommerce refers to using AI, computing, mathematical, and statistical techniques for retail data processing. It involves collecting large volumes of information generated by customer interactions. Thanks to these insights, eCommerce businesses may enhance client journeys and increase their sales.
According to Fortune Business Insights, the retail analytics market size in 2020 was $5.20 billion. From 2021 to 2028, the approximate CAGR (compound annual growth rate) is 17.7%. Also, Mordor Intelligence estimated that the retail big data analytics market will increase at a CAGR of 21.20% in 2023-2027.
In 2020, retail analytics was mostly used for customer management. Fortune Business Insights predicted that this segment would increase its share by 2028. The main reason behind it lies in the consumer demand shift. Thus, many businesses, including eCommerce ones, adapt to changes using data science.
Businesses often rely on Python specialists’ expertise to implement data science solutions. For example, companies can hire Flask developers (either in-house or through outsourcing and IT staffing agencies) to deploy machine-learning models. As a result, they can get APIs and web apps with data science functionalities.
How Can Businesses Leverage Data Science for Increased Consumer Satisfaction?
Customer Service Improvement
Businesses often gather information from surveys, reviews, and social media posts. They can optimize customer feedback analysis using data science in eCommerce. By understanding their clients’ sentiments, companies can promptly identify and address negative experiences.
This technology also helps to determine buyers’ preferences and pain points. As a result, data science insights allow businesses to personalize customer interactions. For instance, each client can get tailored recommendations and relevant content. Customization leads to a more engaging and satisfying experience, strengthening visitors’ loyalty.
Airbnb uses data science to identify patterns in guest and host reviews. Using NLP, it conducts sentiment analysis to gauge customer satisfaction levels. Airbnb can then deal with recurring issues and make improvements in their services.
Clients Behavior Analysis
Identifying trends in customer behavior is a valuable strategy for enhancing client experience. By using eCommerce data, businesses can identify patterns. For instance, they can examine frequently purchased items or preferred categories. This information enables companies to offer targeted promotions. Also, they can look over the browsing behavior and determine common issues.
Data analysis and predictive modeling techniques make it possible to forecast customer needs. In particular, their purchase history helps predict a client’s most exciting products or services. The order date helps to understand when there is a need for replenishment. Thus, organizations can reach out to shoppers with relevant offers proactively.
Netflix uses data science to analyze the relationship between the quality of experience (QoE) and user behavior. For example, they monitor how playback interruption is associated with low bitrate. This popular streaming service then adjusts its algorithms accordingly. Hence, viewers can enjoy the movies, and Netflix can ensure customer satisfaction.
Tailored Recommendations
Businesses can leverage eCommerce data science and machine learning algorithms for product suggestions. They can gather information from clients’ wishlists, browsing, and order history. Then, online stores can consistently offer relevant and appealing item referrals.
These tailored recommendations increase the likelihood of customers purchasing products. Companies can also encourage repeat purchases. Moreover, making customers feel understood strengthens their loyalty to the brand.
Amazon’s RBS (recommendation-based system) is a prominent data science use case. This system seeks and predicts the “rating” a customer would give to an item. The company also tracks user data such as search behavior, address, income level, and so on. Thus, this online retailer considers all factors that might impact the buyers’ decision-making. As a result, Amazon increases sales by offering relevant products to shoppers.
Price Optimization
Data science in eCommerce helps companies make informed decisions on pricing changes. With data analysis tools, organizations can track competitors’ pricing strategies. Based on their insights, they can then adjust their market positioning accordingly.
Also, businesses can determine the price sensitivity of different customer segments. They can use dynamic strategies, such as demand-based or personalized pricing. This data-driven approach enables sales maximization and client satisfaction.
For example, the leading eCommerce platform Alibaba uses data science to optimize prices on its online marketplaces. The company makes adjustments based on supply and demand levels. These informational findings also include sales data and customer preferences. This approach enables Alibaba sellers to attain a competitive edge in terms of pricing.
Inventory Management
Businesses can integrate eCommerce predictive analytics using data science, AI, and machine learning. These advanced techniques enable companies to forecast market trends based on customer data. In particular, online stores can anticipate customer needs and optimize their production planning.
Precise demand prediction helps businesses avoid running out of stock and excess inventory. Thus, they reduce carrying costs, prevent loss of sales, and improve their operational efficiency.
Stitch Fix, a fashion-focused company, leverages data science to enhance inventory management. The company analyzes customer preferences, browsing behavior, and order history. Based on the findings, Stitch Fix comes up with a diverse and curated selection of fashion items. This way, it can predict the level of needed inventory.
Supply Chain Optimization
By using data science in supply chain management, businesses can analyze vast amounts of delivery and logistics data. In particular, routes, weather conditions, and customer locations greatly impact productivity. eCommerce analysis of data helps identify the most efficient delivery paths and optimize their distribution.
The use of data science enhances their fulfillment processes. Data-driven insights help organizations identify bottlenecks in order processing. Hence, they can streamline operations, reduce errors, and expedite delivery times.
Amazon truly understands the importance of data analytics in eCommerce. By leveraging this technology, the company minimizes transportation costs and boosts delivery speed. In fact, Amazon managed to decrease its time from click to ship by 225%.
Conclusion
Data science in eCommerce revolutionizes the way businesses understand and serve their clients. Companies can personalize their shoppers’ experiences, enhance product availability, and streamline their operations. The data science use cases showed the advantages gained in today’s competitive landscape. All in all, customer satisfaction is crucial in the emerging eCommerce world, isn’t it?